SPUR (2024) 7 (2): https://doi.org/10.18833/spur/7/2/3
The dual mission of the Council of Undergraduate Research, to both stimulate the rigorous assessment of undergraduate research programs and encourage models of undergraduate research programs, presents challenges for assessment. This commentary observes the directions taken by assessment when pursuing either a theoretical model of undergraduate research or a role model of undergraduate research. The first direction suggests the goal of generalizable findings afforded by sophisticated quantitative methods. The second direction suggests the goal of transferable programs evaluated with simpler approaches including mere description, graphical presentation, and the evaluation of ostensible confounding variables as support factors for the success of the program. The diversity of undergraduate research and creative inquiry programs points toward the use of student self-disclosures as direct measures of the student experience.
Lopatto, David. 2023. Quantitative Methods in the Assessment of Undergraduate Research, Scholarship, and Creative Inquiry. Scholarship and Practice of Undergraduate Research 7 (2): 5-12. https://doi.org/10.18833/spur/7/2/3
SPUR represents the philosophy of the Council on Undergraduate Research (CUR) to support and promote high-quality mentored undergraduate research, scholarship, and creative inquiry. Readers of SPUR approach the journal hoping to find model programs, good ideas, and the characteristics or causes of successful undergraduate research programs. The dual goals of SPUR, according to LaPlant (2017), are to “stimulate the rigorous assessment of undergraduate research initiatives and programs” and “that SPUR will encourage best practices and models of undergraduate research” (3) Consideration of these goals leads to two views of the use of quantitative methods. Whereas rigorous assessment evokes ideas concerning statistical comparison and control of confounding variables to clarify a theory of undergraduate research, scholarship, and creative inquiry (URSCI) and its effects on student behavior, finding model programs suggests that programs may be emulated across institutions by educators who are free to change support factors to facilitate the program’s success. In the first case the goal is generalizability; in the second the goal is transferability.
The focus of this commentary is on the use of quantitative research methods in the understanding and assessment of undergraduate research, scholarship, and creative inquiry. The Council on Undergraduate Research has a broad definition of undergraduate research (a mentored investigation or creative inquiry conducted by undergraduates that seeks to make a scholarly or artistic contribution to knowledge), meant to include all scholarly disciplines and interdisciplines. It is necessary to acknowledge that disciplinary pluralism implies epistemological pluralism. The assessment of some undergraduate research programs may rely on the qualitative methods suitable for the nature of the program. For example, Naepi and Airini (2019) described the Knowledge Makers program used to mentor Indigenous researchers and evaluated the impact of the program through e-portfolios and student reflections without the use of quantitative data. Zhen (2020) described a program for teaching chefs to be researchers and presented a summary of successful projects as well as a sample of visual evidence (a photograph) in support of the program’s effectiveness. The observations regarding quantitative methods that follow are not intended to privilege quantitative methods over other epistemologies.
Finding model (exemplary) programs suggests that the authors of many of this journal’s reports are advocating causes (the URSCI mission) as well as investigating causes. CUR’s mission attracts the interest of teacher-scholars whose strategic aim is not one of complete disinterest or impartiality. They want the promise of undergraduate research to succeed. SPUR reports are often composed by mentors, instructors, and program directors who have a stake in the success of their program and the broader mission of CUR. The challenge is to practice impartiality when analyzing undergraduate research programs, and it is in this endeavor that quantitative methods may help. Quantitative methodology comprises conventions for best practices that enhance credibility, such as rules applying to the size and scope of an adequate sample and decision rules for what constitutes “statistical significance.” The promise of quantitative methods is that they permit tactics for evaluation that are objective employed by teacher-scholars who have objectives.
Although textbooks about quantitative methods suggest that a research plan should precede the selection of appropriate measures, it appears that educational researchers rely on available measures of program effectiveness such as student grade point average (GPA), graduation rates, or completion rates. The advantages and disadvantages of these measures are familiar. Institutional measures such as the GPA are routinely collected, and archives are readily available. Although most researchers recognize that grade point averages comprise a heterogeneous mix of course selection, degree of difficulty, and other confounds, the archive continues to be employed in assessment and evaluation (Brown et al. 2020; Nicols-Grinenko et al. 2017; Sell, Naginey, and Stanton 2018). In this selection of a measure, familiarity breeds contentment. Reliance on one imperfect measure is a risky method; however, there is a remedy. The methodology of multioperationalism (Cook and Campbell 1979; Webb et al. 1981) suggests that multiple measures may align to support the argument for the benefits of an URSCI program. Therefore analyzing the GPA and another measure such as student survey responses may strengthen the argument for program effectiveness.
Research Questions
The dual targets of CUR’s mission suggest dual research goals. Consistent with the rigorous assessment of undergraduate research initiatives, Haeger et al. (2020) suggest that the key research question for the study of URSCI is to explicate the causal relationship between URSCI and the various outcomes that have been attributed to the experience (e.g., Lopatto 2004). Haeger et al. observe that quantifying the effects of URSCI has been a challenge, writing that “the majority of research measuring the impact of undergraduate research relies on indirect measures or correlations between outcomes and participation” (67) SPUR also promotes the sharing of models of undergraduate research, inviting the transfer of a model program to new settings even though the underlying causal model is not known. Causal models and model programs are not the same and may afford different sorts of quantitative analysis.
Simplicity
When deploying quantitative methods for purposes of describing or evaluating URSCI, it is tempting to bring to bear the full persuasive impact of sophisticated methods used to uncover latent variables, account for more multiple factors and their interactions, and seek an elusive generalizability of the pedagogy’s effects. There is value in choosing a more modest approach. Experienced researchers caution us that “less is more.” Cohen (1990), writing about psychology, advocated simplicity in research designs, citing the problems that accompany complexity, including poor statistical power (the probability of finding an effect if it exists) and the increase in misleading conclusions of statistical significance when the number of tests increases. Kass et al. (2016) included “keep it simple” in their advice regarding effective statistical practice in computational biology. They wrote, “the principle of parsimony can be a trusted guide: start with simple approaches and only add complexity as needed, and then only add as little as seems essential” (4) Abelson (1995) wrote, “Data analysis should not be pointlessly formal. It should make an interesting claim . . . and do so by intelligent interpretation of appropriate evidence from empirical measurements or observations.” In support of interesting claims authors often use familiar quantitative methods even if the disciplinary focus of the undergraduate research program is complex. For example, the SPUR issue for summer 2019 highlighted programs that featured undergraduate research experiences using big data (large databases and data visualization). None of the featured programs employed big data techniques to evaluate the program’s outcomes. Some reports favored descriptive statistics (Killion, Page, and Yu 2019; Lukes et al. 2019). Others favored descriptions of the program’s development or evolution without quantitative evaluation (Nelson, Yusef, and Cooper 2019). It may be that even as URSCI programs grow to embrace contemporary topics such as machine learning, digital humanities, and artificial intelligence the quantitative methods by which the programs are assessed remain relatively simple. Simple analyses include the t test, which was intended for samples smaller than 30 when comparing a treatment group to a comparison group; as well there is a version for pretest-posttest comparisons. Some reports (e.g., McLaughlin, Patel, and Slee 2020) employ nonparametric statistics that do not demand normally distributed data. Faced with small samples of less than 30, some reports acknowledge the difficulty of inferential comparisons and report only descriptive statistics (Dillon 2020; Spronken-Smith et al. 2018). For these small groups visual representation of data is helpful. If a cohort of students engaging in program is very small, then it may be important to note the reasons for any student who fails to benefit or who drops out of the program. These reasons may be exogenous to the program, such as illness, family crises, etc., and so may not influence the argument for the program’s effectiveness.
Description
Quantitative data are the most common form of reporting the results of programs described in SPUR and other journals; however, the reliance on data does not compel inferential testing or model building. Instead, numerical data can be used as “mere description” (Gerring 2012), providing a more precise account of outcomes than qualitative summaries. If a study reports that student program participants average grades of 3.7, most readers know implicitly that the common GPA scale ranges from 0 to 4, and that 3.7 is a successful grade. Deming (1953) distinguished between surveys that were enumerative (asking how much) and surveys that were analytic (asking why). Enumerative surveys may be adequate for evaluating the effectiveness of a program by reporting graduation rates or attrition rates, vouching for the success of the program but falling short of specifying the specific cause of the success (Cartwright 2007). In some studies mere description is adequate for illustrating an effect. For example, Grindle et al. (2021) used descriptive counts and percentages to illustrate the result of in a study of passive research involvement.
Cohen (1990) noted that simplicity of describing data suggests the use of graphs and diagrams that may aid in presenting a program outcome. The use of figures may efficiently represent descriptive data, and is a common practice in this journal (Barney 2017; Brooks et al. 2019; Garrett et al. 2021; Gold, Atkins, and McNeal 2021; Kuan and Sedlacek 2022; Szecsi et al. 2019). Tufte (1983) outlined the characteristics of graphical excellence, including graphs that serve the clear purpose of description, which encourage the viewer to think about substance, and encourage comparisons between different pieces of data.
Validity
Readers expect the assessment of an URSCI program to be valid. There are many adjectives to be placed before validity, and inevitably three types occur. The first is the validity of the instruments employed to measure outcomes. The second has to do with the internal logic of the program and how that program produces results (internal validity). Finally, the question of the generalizability or transferability of the program arises (external validity).
We expect the creators of instruments to present some evidence of the instrument’s validity by showing that the instrument is in agreement with other methods used to measure the same outcome (Campbell and Fiske 1959). Once the instrument’s trustworthiness is established, the use of the instrument by subsequent researchers often relies on the reputation of the instrument’s original validation. There is often not enough data or time to revisit techniques for validation of the instrument in every study. This trust in the instrument is normal; work proceeds slowly if the research instrument has to be revalidated each time. The concern arises when the new users of the instrument invoke a “mutatis mutandis” approach, that is, making necessary changes in the original instrument so that it fits the new project without affecting the main constructs measured by the instrument. The presumption is that the original instrument is robust, preserving its validity despite alterations. A perusal of the reports published in SPUR suggests that authors often use research instruments created by other researchers. Examples include the SURE survey (Survey of Undergraduate Research Experiences; Lopatto 2004); URSSA (Undergraduate Research Student Self-Assessment; Hunter et al. 2009); and the OSCAR Student Survey (Foster and Usher 2018). Items from these established surveys are occasionally revised to suit the context. Are there credible procedures for changing an instrument while claiming that it retains its essential meaning? The credibility of the instrument can be supported by response process validity, which involves the review of the survey items by subject matter experts, and cognitive interviewing of potential respondents to determine if respondents understood the intended meaning of the survey items. These procedures may or may not lend themselves to quantitative analysis, but they improve the validity of the modified instrument.
The effectiveness of the program, called internal validity, is “the degree to which an experiment is methodologically sound and confound-free” (Goodwin and Goodwin 2017, 148). The validity question reduces to the confidence we have that the URSCI program causes the changes in the students’ behaviors. Traditionally, the gold standard for causal assertions is the true experiment, or randomized controlled trial. Randomized controlled trials are rare in studies of undergraduate research and creativity. Randomized controlled trials rely on the researcher’s control of participant assignment to treatment and comparison groups as the basis for making a causal assertion that the program caused changes in the participant’s behavior. In the absence of randomized controlled trials design features for a causal assertion, researchers use a variety of tactics. Some involve the creation of a nonequivalent comparison group that serves as a proxy for a genuine control group. Nicols-Grinenko et al. (2017) utilized their institution’s undergraduate population as a comparison group for students who participated in undergraduate research. After describing an initiative to build a culture of undergraduate research at their institution, they tracked undergraduate research participants and compared the participants graduation rates and grade point averages to all undergraduate contemporaries. They found higher graduation rates and grade point averages for undergraduate research participants compared to the general student population. Several researchers use a pretest as the comparison group for posttest data. Beer et al. (2019) used both between-groups and pretest-posttest data to argue for the effectiveness of a peer research consultant program. The results showed increments in desirable skills from pretest to posttest based on t tests. Ashcroft et al. (2021) employed pre- and post-ratings of gains in the understanding of research and related items and found several significant Wilcoxon test results in the favorable direction. Tian et al. (2022) reported on the success of inquiry-based learning in China. They found significant gains on self-report items from the SURE survey (Lopatto 2004), although the choice of inferential test was unclear. Several of these reports chose to analyze items on a survey separately, leading to the concern that piecemeal testing may result in false positives (type 1 errors).
Matching and pretest-posttest designs are efforts to preserve the internal validity of the assessment in the absence of experimental control. The objective is a generalizable result. The most ambitious attempts to substitute statistical control for experimental control involve forms of multiple regression models.
Models
The term model can be used to describe a “particular aspect of a given theory” (Fried 2020) or a program to be emulated. In the model as theory, the undergraduate research program is described for replication with adherence to the original method, that is, the program is generalizable. The model as theory suggests that the reader will see a SPUR report that describes an outcome for a sample (usually of undergraduate students) that will generalize to a population. Because URSCI programs seldom follow the formula for assertions of generalization, namely, randomly selecting student participants from the student population and randomly assigning students to treatment and control groups (see Haeger et al. 2020), researchers exploring the nature of undergraduate research employ various statistical methods as a substitute for randomization. The goal is to estimate the main effects of the program to build a theory of URSCI. Student participants in these programs tend to be diverse and so confound the main effects of the program. How do researchers attempt to account for student differences? Some analyses of undergraduate research (UR) include attempts at matching non–randomly assigned program participants with nonparticipants. These analyses employ a range of techniques from simple matching to advanced regression analysis to examine whether student characteristics moderated the program outcome. Rodenbusch et al. (2016), for example, reported that regression analysis of race/ethnicity, gender, and first-generation undergraduate status yielded no significant relation to program success. Galli and Bahamonde (2018) matched UR students and comparison groups on grade point average at time of program admission. Whittinghill et al. (2019) reported an analysis of 10 years of data concerning the effect of UR on graduate rates, grade point average, and entrance into graduate programs. They used propensity matching (Rosenbaum and Rubin 1983) to create a quasi-control group for comparison with the outcomes for UR researchers. Brouhle and Graham (2022) employed a probit regression model to account for possible confounding variables affecting undergraduate research students and a comparison group of nonresearchers. The technique allowed the researchers to argue that differential outcomes, such as the superior grade point averages of the undergraduate research students, were not based on a confounding variable. Sell, Naginey, and Stanton (2018) compared the grade point averages of students with research experience with those who did not, for both contemporary students and graduates. For graduates, propensity matching was used to form a matched comparison group to the undergraduate research group. The analysis, which matched the groups on eight variables including gender and first-generation undergraduate status, found significantly higher grade point averages for research students.
Large-scale programs, or programs that consolidate data over several years, recognize that the student is a heterogenous variable, that is, within the student sample there are many subsamples. These subsamples may be classified by race, ethnicity, gender, or culture. Large-scale programs intend to benefit all students, so quantitative methods are employed to show how well the program results in a general main effect. Some large-scale programs test for differences between student subsamples on a quantitative measure and simply report that no differences were found (Shaffer et al. 2014). Others use sophisticated modeling to eliminate the influence of possible confounds. Hanauer et al. (2017) examined the impact of the SEA-PHAGES undergraduate research program in biology on student success while accounting for a variety of student characteristics. They reported equally positive outcomes for students with diverse economic backgrounds, academic performance, gender, and ethnicity. The intent of these approaches is that they attempt to preserve the idea of the general reference population, that demographic and economic identities of students are confounding variables that may be removed from the analysis statistically, revealing a main effect of URSCI on the general reference population of undergraduate students.
The third use of validity is external validity, usually defined as the degree to which research findings generalize to other populations, settings, or times. The usual argument is that the results drawn from a sample generalize to a reference population. The construct of the reference population to which studies generalize has been questioned by awareness of how WEIRD (Western, educated, industrialized, rich, and democratic) cultural participants in psychological research skew the results away from generalizability (American Psychological Association 2010). Reports published in SPUR seem cognizant of the need to address multiple student populations, an approach sometimes termed culturally responsive assessment (Baker and Henning 2022). Pursuing the goal of generalizability encourages analysts to control confounding variables such as student ethnicity or gender. Pursuing the goal of transferability encourages the consideration of these variables as support factors that are not neutralized but optimized to promote student success. Following Cartwright and Hardie (2012), researchers should be free to optimize support factors rather than to suppress confounding variables. Support factors are “other members of the team of causes” that optimize success. For example, reported successes for undergraduate research in genomics (Lopatto et al. 2008) originated at an institution known for high student selectivity and good financial resources. Reported success of the same program at community colleges (Croonquist et al. 2023) required the recognition that many support factors of the community college programs differed from those in the early reports. Further examples of diverse yet effective programs may be found in the SPUR special issue published in summer 2018, which highlighted culturally relevant programs (Boudreau et al. 2018; Puniwai-Ganoot et al. 2018) that reported effectiveness without claiming to be replications of a standard method. Each program deployed a package of support factors to optimize the program’s success. Whereas studies in pursuit of generalizable results set aside variables such as gender, ethnicity, and socioeconomic status, culturally relevant programs foreground these variables and employ the necessary support factors to facilitate the program’s, and the student’s, success. SPUR reports often suggest model programs that may be emulated (Dickter et al. 2018; Follmer et al. 2017; Foster and Usher 2018; Gilbertson et al. 2021; Gould 2018). The approach makes sense, given that SPUR is a trading post of ideas across academic disciplines and interdisciplines.
SPUR and its parent organization CUR value diversity and equity. Equity is typically taken to mean that different students need adjustments to correct for imbalances and obstacles to success. Equity is a support factor. Equity adjustments imply that students are not replicates of each other. The challenge, then, is to find measures of program effectiveness that includes the individual differences of student participants. For this purpose, it is necessary to reimagine a common distinction in assessment research between direct and indirect measures of student behavior. Direct measures of student learning are said to include tests of knowledge such as exams and quizzes. Indirect measures of student learning include quantitative self-reports found in surveys. Although the multioperational approach to assessment (Cook and Campbell 1979) recommends the use of both measures rather than relying on one, direct measures have been enshrined as superior to student self-reported measures. Within URSCI programs the privileged status of direct measures needs to be interrogated, given that many programs encourage students to create unique products, artifacts, or scholarly reports. The interrogation may proceed in this way: Indirect measure of student behavior, that is, self-reported quantitative ratings, seem to cast the student as an audience to some instructional performance. The self-report is often anonymous, preventing the appreciation of the role of the student’s identity in their experience. In undergraduate research, scholarship, and creative inquiry the student is an active participant (but see Grindle et al. 2021). Their experience is necessarily interpreted through the lens of their personal identity. URSCI experiences may modify or enlarge the student’s identity with respect to professionalism or joining a community of scholars (Palmer et al. 2018). Rigorous statistical modeling treats aspects of identity as confounding variables that need to be partitioned from the main effect of URSCI so that a generalizable treatment effect may be uncovered. Standard quantitative methods such as analysis of variance or multiple linear regression treat the interaction of the independent variable and the student’s identity as an isolatable, additive, and linear component of the experience. If the goal of the assessment is not, however, a generalization from the student sample to a unitary reference population, then we may become interested in the student’s identity as a support factor for the program’s success. The joint effect of a program and the student’s identity is not an interaction but an intersection. The individual differences of the students become a focus of assessment, and the student’s survey data evolves from indirect measure to direct measure. Self-report becomes self-disclosure. Self-disclosure offers the most direct measure of the student’s URSCI experience. The challenge going forward is to optimize the use of quantitative methods to find precise descriptors of student outcomes while preserving the individual differences in student success.
The continuing challenge for faculty and staff who administer undergraduate research programs will be the nearly compulsory assessment of student learning and attitude. The work may seem challenging to program faculty and staff who do not regularly employ quantitative methods. Consulting the myriad online courses, websites, and videos concerning statistics may be off-putting. A less abrasive introduction to quantitative methods may be sources such as Statistics Done Wrong (Reinhart 2015) or Statistics As Principled Argument (Abelson 1995), books that address common problems of quantitative decision-making without elaborate formulas. Similarly, The Craft of Research (Booth et al. 2016), although it does not cover statistical analysis, has a useful chapter on communicating evidence visually. For readers wishing to tutor themselves in statistical techniques there are Statistics Unplugged (Caldwell 2013) and Statistics for the Terrified (Kranzler 2003). For issues concerning quasi-experimental design and threats to validity, Cook and Campbell (1979) remains a standard text.
Encouraging best practices includes encouraging the practitioner. The ongoing explorations in programs for undergraduate research, scholarship, and creative inquiry will best be sustained if they are beneficial to the student and the mentor. Quantitative methods may provide a perspective through which the benefits may be discerned. The construction of this perspective and the picture that emerges provide a shared journey for all participants.
Conflict of Interest
The author has no conflict of interest.
IRB Statement
Not applicable.
Data Availability
Not applicable.
References
Abelson, Robert P. 1995. Statistics As Principled Argument. Hillsdale, NJ: Lawrence Erlbaum.
American Psychological Association. 2010. “Are Your Findings ‘WEIRD’?” Monitor on Psychology 41(5): 11. Accessed July 17, 2023. https://www.apa.org/monitor/2010/05/weird
Ashcroft, Jared, Veronica Jaramillo, Jillian Blatti, Shu-Sha Angie Guan, Amber Bui, Veronica Villasenor, Alina Adamian, et al. 2021. “BUILDing Equity in STEM: A Collaborative Undergraduate Research Program to Increase Achievement of Underserved Community College Students.” Scholarship and Practice of Undergraduate Research 4(3): 47–58. doi: 10.18833/spur/4/3/11
Baker, Gianina R., and Gavin W. Henning. 2022. “Current State of Scholarship on Assessment.” In Reframing Assessment to Center Equity: Theories, Models, and Practices, ed. Gavin Henning, Gianina R. Baker, Natasha A. Jankowski, Anne E. Lundquist, and Erick Montenegro, 57–79. Sterling, VA: Stylus.
Barney, Christopher C. 2017. “An Analysis of Funding for the NSF REU Site Program in Biology from 1987 to 2014.” Scholarship and Practice of Undergraduate Research 1(1): 11–19. doi: 10.18833/spur/1/1/1
Beer, Francisca, Christina M. Hassija, Arturo Covarrubias-Paniagua, and Jeffrey M. Thompson. 2019. “A Peer Research Consultant Program: Feasibility and Outcomes.” Scholarship and Practice of Undergraduate Research 2(3): 4–13. doi: 10.18833/spur/2/3/4
Booth, Wayne C., Gregory G. Colomb, Joseph M. Williams, Joseph Bizup, and William T. Fitzgerald. 2016. The Craft of Research. 4th ed. Chicago: University of Chicago Press.
Boudreau, Kristin, David DiBiasio, and Zoe Reidinger. 2018. “Undergraduate Research and the Difference It Makes for LGBTQ+ Students.” Scholarship and Practice of Undergraduate Research 1(4): 46–47. doi: 10.18833/spur/1/4/1
Brooks, Andrea Wilcox, Jane Hammons, Joseph Nolan, Sally Dufek, and Morgan Wynn. 2019. “The Purpose of Research: What Undergraduate Students Say.” Scholarship and Practice of Undergraduate Research 3(1): 39–47. doi: 10.18833/spur/3/1/7
Brouhle, Keith, and Brad Graham. 2022. ”The Impact of Undergraduate Research Experiences on Graduate Degree Attainment across Academic Divisions.” Scholarship and Practice of Undergraduate Research 6(1): 32–42.
Brown, Daniel A., Nina B. Wright, Sylvia T. Gonzales, Nicholas E. Weimer, and Julio G. Soto. 2020. “An Undergraduate Research Approach That Increased Student Success at a Hispanic-Serving Institution (HSI): The SURE Program at Texas State University.” Scholarship and Practice of Undergraduate Research 4(1): 52–62. doi: 10.18833/spur/4/1/18
Caldwell, Sally. 2013. Statistics Unplugged. 4th ed. Belmont, CA: Wadsworth, Cengage Learning.
Campbell, Donald T., and Donald W. Fiske. 1959. “Convergent and Discriminant Validation by the Multitrait-Multimethod Matrix.” Psychological Bulletin 56: 81–105.
Cartwright, Nancy. 2007. Hunting Causes and Using Them: Approaches in Philosophy and Economics. Cambridge, UK: Cambridge University Press.
Cartwright, Nancy, and Jeremy Hardie. 2012. Evidence-Based Policy: A Practical Guide to Doing It Better. Oxford, UK: Oxford University Press.
Cohen, Jacob. 1990. “Things I Have Learned (So Far).” American Psychologist 45: 1304–1312.
Cook, Thomas D., and Donald T. Campbell. 1979. Quasi-Experimentation: Design and Analysis Issues for Field Settings. Boston: Houghton Mifflin.
Croonquist, Paula, Virginia Falkenberg, Natalie Minkovsky, Alexa Sawa, Matthew Skerritt, Maire K. Sustacek, Raffaella Diotti, et al. 2023. “The Genomics Education Partnership: First Findings on Genomics Research in Community Colleges.” Scholarship and Practice of Undergraduate Research 6(3): 17–28. doi: 10.18833/spur/6/3/1
Deming, W. Edward. 1953. “On the Distinction between Enumerative and Analytic Surveys.” Journal of the American Statistical Association 48: 244–255.
Dickter, Cheryl L., Anne H. Charity Hudley, Hannah A. Franz, and Ebony A. Lambert. 2018. “Faculty Change from Within: The Creation of the WMSURE Program.” Scholarship and Practice of Undergraduate Research 2(1): 24–32. doi: 10.18833/spur/2/1/6
Dillon, Heather E. 2020. “Development of a Mentoring Course-Based Undergraduate Research Experience (M-CURE).” Scholarship and Practice of Undergraduate Research 3(4): 26–34. doi: 10.18833/spur/3/4/7
Follmer, D. Jake, Sarah Zappe, Esther Gomez, and Manish Kumar. 2017. “Student Outcomes from Undergraduate Programs: Comparing Models of Research Experiences for Undergraduates (REUs).” Scholarship and Practice of Undergraduate Research 1(1): 20–27. doi: 10.18833/spur/1/1/5
Foster, Stephanie L., and Bethany M. Usher. 2018. “Comparing Two Models of Undergraduate Research Using the OSCAR Student Survey.” Scholarship and Practice of Undergraduate Research 1(3): 30–39. doi: 10.18833/spur/1/3/6
Fried, Eiko I. 2020. “Theories and Models: What They Are, What They Are For, and What They Are About.” Psychological Inquiry 31: 336–344. doi: 10.1080/1047840X.2020.1854011
Galli, Dominique M., and Rafael Bahamonde. 2018. “Assessing IUPUI’s Diversity Scholars Research Program: Lessons Learned.” Scholarship and Practice of Undergraduate Research 1(4): 12–17. doi: 10.18833/spur/1/4/10
Garrett, Arnell, Frances D. Carter-Johnson, Susan M. Natali, John D. Schade, and Robert M. Holmes. 2021. “A Model Interdisciplinary Collaboration to Engage and Mentor Underrepresented Minority Students in Lived Arctic and Climate Science Research Experiences.” Scholarship and Practice of Undergraduate Research 5(1): 16–26. doi: 10.18833/spur/5/1/4
Gerring, John. 2012. “Mere Description.” British Journal of Political Science 42: 721–746.
Gilbertson, Lynn, Jeannine Rowe, Yeongmin Kim, Catherine W. M. Chan, Naomi Schemm, and Michael Unhoch. 2021. “An Online Training Program to Enhance Novice Researchers’ Knowledge and Skills.” Scholarship and Practice of Undergraduate Research 4(4): 33–41. doi: 10.18833/spur/4/4/4
Gold, A. U., Rachel Atkins, and Karen S. McNeal. 2021. “Undergraduates’ Graph Interpretation and Scientific Paper Reading Shift from Novice- to Expert-Like as a Result of Participation in a Summer Research Experience: A Case Study.” Scholarship and Practice of Undergraduate Research 5(2): 8–19. doi: 10.18833/spur/5/2/2
Goodwin, Kerri A., and C. James Goodwin. 2017. Research in Psychology: Methods and Design. Las Vegas, NV: Wiley.
Gould, Laurie. 2018. “Introduction: Models of Undergraduate Research Mentoring.” Scholarship and Practice of Undergraduate Research 2(1): 2–3. doi: 10.18833/spur/2/1/10
Grindle, Nicholas, Stefanie Anyadi, Amanda Cain, Alastair McClelland, Paul Northrop, Rebecca Payne, and Sara Wingate Gray. 2021. “Re-Evaluating Passive Research Involvement in the Undergraduate Curriculum.” Scholarship and Practice of Undergraduate Research 5(1):52–58. doi: 10.18833/spur/5/1/12
Haeger, Heather, John E. Banks, Camille Smith, and Monique Armstrong-Land. 2020. “What We Know and What We Need to Know about Undergraduate Research.” Scholarship and Practice of Undergraduate Research 3(4): 62–69. doi: 10.18833/spur/3/4/4
Hanauer, David I., Mark J. Graham, SEA-PHAGES, Laura Betancur, Aiyana Bobrownicki, Steven G. Cresawn, Rebecca A. Garlena, et al. 2017. “An Inclusive Research Education Community (iREC): Impact of the SEA-PHAGES Program on Research Outcomes and Student Learning.” Proceedings of the National Academy of Sciences 114: 13531–13536. doi: 10.1073/pnas.1718188115
Hunter, Anne-Barrie, Timothy Weston, Sandra L. Laursen, and Heather Thiry. 2009. “URSSA: Evaluating Student Gains From Undergraduate Research in the Sciences.” CUR Quarterly 29(3): 15–19.
Kass, Robert E., Brian S. Caffo, Marie Davidian, Xiao-Li Meng, Bin Yu, and Nancy Reid. 2016. “Ten Simple Rules for Effective Statistical Practice.” PLOS Computational Biology 12(6): e1004961. doi: 10.1371/journal.pcbi.1004961
Killion, Patrick J., Ian B. Page, and Victoria Yu. 2019. “Big-Data Analysis and Visualization as Research Methods for a Large-Scale Undergraduate Research Program at a Research University.” Scholarship and Practice of Undergraduate Research 2(4): 14–22. doi: 10.18833/spur/2/4/7
Kranzler, John H. 2003. Statistics For The Terrified. 3rd ed. Upper Saddle River, NJ: Pearson Education.
Kuan, Jennifer, and Quentin C. Sedlacek. 2022. “Does It Matter If I Call It a CURE? Identity Development in Online Entrepreneurship Coursework.” Scholarship and Practice of Undergraduate Research 6(1): 23–31. doi: 10.18833/spur/6/1/7
LaPlant, James T. 2017. “Welcome to the Inaugural Issue of SPUR.” Scholarship and Practice of Undergraduate Research 1(1): 3–4.
Lopatto, David. 2004. “Survey of Undergraduate Research Experiences (SURE): First Findings.” Cell Biology Education 3: 270–277. doi: 10.1187/cbe.04-07-0045
Lopatto, David, Consuelo Alvarez, Daron Barnard, Chitra Chandrasekaran, Hui-Min Chung, Charles Du, Todd Eckdahl, et al. 2008. “Genomics Education Partnership.” Science 322: 684–685. doi: 10.1126/science.1165351
Lukes, Laura A., Katherine Ryker, Camerian Millsaps, Rowan Lockwood, Mark D. Uhen, Christian George, Callan Bentley, and Peter Berquist. 2019. “Leveraging a Large Database to Increase Access to Undergraduate Research Experiences.” Scholarship and Practice of Undergraduate Research 2(4): 4–13. doi: 10.18833/spur/2/4/6
McLaughlin, Jacqueline S., Mit Patel, and Joshua B. Slee. 2020. “A CURE Using Cell Culture–Based Research Enhances Career-Ready Skills in Undergraduates.” Scholarship and Practice of Undergraduate Research 4(2): 49–61. doi: 10.18833/spur/4/2/15
Naepi, Sereana, and Airini. 2019. “Knowledge Makers: Indigenous Student Undergraduate Researchers and Research.” Scholarship and Practice of Undergraduate Research 2(3): 52–60. doi: 10.18833/spur/2/3/7
Nelson, Randy B., Kideste Mariam Yusef, and Adrienne Cooper. 2019. “Expanding Minds through Research: Juvenile Justice and Big Data.” Scholarship and Practice of Undergraduate Research 2(4): 30–36. doi: 10.18833/spur/2/4/10
Nicols-Grinenko, Annemarie, Rachel B. Verni, Jennifer M. Pipitone, Christin P. Bowman, and Vanya Quinones-Jenab. 2017. “Building a Culture of Undergraduate Research: A Case Study.” Scholarship and Practice of Undergraduate Research 1(2): 43–51. doi: 10.18833/spur/1/2/13
Palmer, Ruth J., Andrea N. Hunt, Michael R. Neal, and Brad Wuetherick. 2018. “The Influence of Mentored Undergraduate Research on Students’ Identity Development.” Scholarship and Practice of Undergraduate Research 2(2): 4–14. doi: 10.18833/spur/2/2/1
Puniwai-Ganoot, Noelani, Sharon Ziegler-Chong, Rebecca Ostertag, and Moana Ulu Ching. 2018. “Mentoring Pacific Island Students for Conservation Careers.” Scholarship and Practice of Undergraduate Research 1(4): 25–32. doi: 10.18833/spur/1/4/11
Reinhart, Alex. 2015. Statistics Done Wrong: The Woefully Complete Guide. San Francisco: No Starch Press.
Rodenbusch, Stacia E., Paul R. Hernandez, Sarah L. Simmons, and Erin L. Dolan. 2016. “Early Engagement in Course-Based Research Increases Graduation Rates and Completion of Science, Engineering, and Mathematics Degrees.” Cell Biology Education–Life Sciences Education 15(2): ar20. doi: 10.1187/cbe.16-03-0117
Rosenbaum, Paul R., and Donald B. Rubin. 1983. “The Central Role of the Propensity Score in Observational Studies for Causal Effects.” Biometrika 70: 41–55. doi: 10.1093/biomet/70.1.41
Sell, Andrea J., Angela Naginey, and Cathy Alexander Stanton. 2018. “The Impact of Undergraduate Research on Academic Success.” Scholarship and Practice of Undergraduate Research 1(3): 19–29. doi: 10.18833/spur/1/3/8
Shaffer, Christopher D., Consuelo J. Alvarez, April E. Bednarski, David Dunbar, Anya L. Goodman, Catherine Reinke, Anne G. Rosenwald, et al. 2014. “A Course-Based Research Experience: How Benefits Change with Increased Investment in Instructional Time.” Cell Biology Education–Life Sciences Education 13: 111–30. doi: 10.1187/ cbe-13-08-0152
Spronken-Smith, Rachel, Sally Sandover, Lee Partridge, Andy Leger, Tony Fawcett, and Liz Burd. 2018. “The Challenges of Going Global with Undergraduate Research: The Matariki Undergraduate Research Network.” Scholarship and Practice of Undergraduate Research 2(2): 64–72. doi: 10.18833/spur/2/2/8
Szecsi, Tunde, Charles Gunnels, Jackie Greene, Vickie Johnston, and Elia Vazquez-Montilla. 2019. “Teaching and Evaluating Skills for Undergraduate Research in the Teacher Education Program.” Scholarship and Practice of Undergraduate Research 3(1): 20–29. doi: 10.18833/spur/3/1/5
Tian, Jing, Yiheng Wang, Ghang Ren, and Yingzhe Lei. 2022. “Undergraduate Research and Inquiry-Based Learning in Geographical Information Science: A Case Study from China.” Scholarship and Practice of Undergraduate Research 5(4): 16–23. doi: 10.18833/spur/5/4/8
Tufte, Edward R. 1983. The Visual Display of Quantitative Information. Cheshire, CT: Graphics.
Webb, Eugene J., Donald T. Campbell, Richard D. Schwartz, Lee Sechrest, and Janet B. Grove. 1981. Nonreactive Measures in the Social Sciences. Boston: Houghton Mifflin.
Whittinghill, Jonathan C., Simeon P. Slovacek, Laura P. Flenoury, and Vivian Miu. 2019. “A 10-Year Study on the Efficacy of Biomedical Research Support Programs at a Public University.” Scholarship and Practice of Undergraduate Research 3(1): 30–38. doi: 10.18833/spur/3/1/3
Zhen, Willa. 2020. “Teaching Research Skills to Vocational Learners: Teaching Chefs to Research.” Scholarship and Practice of Undergraduate Research 4(2): 21–26. doi: 10.18833/spur/4/2/6
David Lopatto
Grinnell College
lopatto@grinnell.edu
David Lopatto is a professor of psychology and the Samuel R. and Marie-Louise Rosenthal Professor of Natural Science and Mathematics at Grinnell College. He is the former director of the Grinnell College Center for Teaching, Learning, and Assessment. He has been studying the features and benefits of undergraduate research experiences for many years, creating instruments, including the Survey of Undergraduate Research Experiences (SURE) and the Survey of Classroom Undergraduate Research Experiences (CURE), which may be found at https://sure.sites.grinnell.edu.
More Articles in this Issue
- Book Review‐ Joseph M. Smith
SPUR (2024) 7 (2): https://doi.org/10.18833/spur/7/2/5 Abstract:Conducting Research with Human Participants is presented as an institutional review board (IRB) guide for students and faculty alike. The format is one of a textbook, with learning objectives at the beginning and a chapter summary at the end of each chapter. Chapter summaries are helpful and succinct. They are followed by “Questions to Ask Yourself” that allow readers to reflect on their own previous and current experiences. Finally, “Concepts in Focus for your IRB Work” provides a further distillation of the topics discussed in the chapter.
Smith, Joseph 2023. Conducting Research with Human Participants: An IRB Guide for Students and Faculty Project… - Article‐ Heather D. Vance-Chalcraft, Randall Etheridge, Michael O’Driscoll, Ariane Peralta, Clark Andersen, Fiona Freeland, Joi P. Walker
SPUR (2024) 7 (2): https://doi.org/10.18833/spur/7/2/9 Abstract:Collaborative teamwork is fundamental to successful research and is a desirable skill set for employers. Yet students receive little training in how to effectively work in teams. This article presents the preliminary design and implementation of course-based undergraduate research experiences (CUREs) in biology, geology, and environmental engineering in which student teams address questions related to their discipline while contributing to a shared research project. Team science training in communication, research planning, and conflict resolution was embedded into CURE classes at a regional R2 university. Although barriers to this approach were present, evidence in the form of writing prompt scores and team science products suggested student understanding of effective teams and the benefits of working with individuals within and across disciplines to solve complex problems increased.
Vance-Chalcraft,Heather, Randall Etheridge, Michael O’Driscoll, Ariane Peralta, Clark Andersen, Fiona Freeland, Joi P. Walker. 2023. Investigating… - Article‐ Sophia Mun
SPUR (2024) 7 (2): https://doi.org/10.18833/spur/7/2/6 Abstract:In this study, the Research Methods Sequence (RMS) courses in the College of Behavioral and Social Sciences at California Baptist University were evaluated. There were two central aims in the study. First, the effectiveness of the RMS courses in developing students’ research skills and perceptions of the research process were assessed. Second, the investigator explored significant factors in the success of research experience in an undergraduate program. It was found that the RMS courses improved students’ proficiency in research-related skills. Students reported gains in thinking and working like a scientist, personal gains related to research work, gains in research skills, and gains in knowledge about and attitude toward research. Furthermore, out-of-course research activities and mentor relationships contributed to the success of the undergraduate research experience.
Mun, Sophia. 2023. Evaluation of Research Experiences for Undergraduate Program in Behavioral Sciences: From the Two-Year… - Article‐ Jenna A. Lamphere, Marissa Palmer, Valerie F. Sloan
SPUR (2024) 7 (2): https://doi.org/10.18833/spur/7/2/8 Abstract:During the COVID-19 pandemic, many undergraduate internships, including the National Science Foundation (NSF) Research Experiences for Undergraduates (REU) programs, were canceled or moved online. Although several studies have examined student success during the online transition, less research has examined how REU programs have changed from this experience, the ongoing and novel challenges, and strategies that program coordinators employed to overcome them. To investigate this gap, REU site programs were surveyed in the NSF Geosciences (GEO) Directorate, with findings that many students declined participation after having been accepted into programs, and that there were difficulties accessing institutional support services and meeting changing student needs. Despite these challenges, nearly all respondents reported program satisfaction, with several indicating the importance of GEO REU community support. Overall, REU coordinator resilience appears to be a major factor in program success.
Lamphere, Jenna, Marissa Palmer, Valerie F. Sloan 2023. NSF GEO REU Program Coordinators Show Adaptability and… - Communication‐ Danica E. White, Erica Mi
SPUR (2024) 7 (2): https://doi.org/10.18833/spur/7/2/4 Abstract:Undergraduate research offers a significant avenue for enhancing the engagement and skill development of underrepresented students, particularly those from BIPOC backgrounds. This study conducted at Penn State University aimed to illuminate the challenges faced by BIPOC students in their pursuit of undergraduate research opportunities and promote diversity and inclusivity within research programs. Through interviews and surveys, the research team discovered that students often grapple with uncertainty when seeking research prospects, with disparities in access to information. They also highlighted the importance of faculty mentorship, particularly from individuals with shared backgrounds. Although opinions on the influence of race or ethnicity on research experiences varied, the study emphasized the need for tailored support and proactive outreach efforts to improve the accessibility of undergraduate research resources.
White, Danica, Erica Mi. 2023. Examining BIPOC Student Barriers in Undergraduate Research. Scholarship and Practice of… - Article‐ Cinthia Pacheco, Amy Hessl, John Campbell, Paige Zalman, Carinna Ferguson
SPUR (2024) 7 (2): https://doi.org/10.18833/spur/7/2/1 Abstract:This article describes the Research Apprenticeship Program (RAP), a mentored undergraduate research experience implemented in 2017 at a public land-grant institution located in the Appalachian region. The article focuses on RAP’s approach to recruiting, retaining, and supporting students in faculty-mentored research and creative inquiry. To assess the impact of RAP on undergraduate retention, institutional data were collected to identify RAP participants from the years 2017 to 2022 (n = 868) to compare next-year retention rates with institutional averages across similar demographic groups. The results showed that retention rates for RAP participants were significantly higher than institutional averages, and disaggregated data also showed higher retention rates for participants from historically marginalized populations. These results provide evidence of the program’s contribution to the educational development of the Appalachian region.
Pacheco, Cinthia, Amy Hessl, John Campbell, Paige Zalman, Carinna Ferguson. 2023. Increasing Undergraduate Retention in Appalachia… - Article‐ Jackie Swanik, Stephanie Rollins, Sarah Horstman, Carolyn Hoffman, Kimberly Fishback
SPUR (2024) 7 (2): https://doi.org/10.18833/spur/7/2/7 Abstract:This study investigates the impact of community college students’ participation in mentored undergraduate research, with an emphasis on STEM transfer students. The STEM Academic Research and Training (START) program at Wake Technical Community College was designed and implemented by community college faculty and staff and was evaluated in a randomized control trial. Early evidence showed statistically significant positive effects on students’ attitudes toward STEM.
Swanik, Jackie, Stephanie Rollins, Sarah Horstman, Carolyn Hoffman, Kimberly Fishback. 2023. Mentored Undergraduate Research at Community… - Open-to-Read‐ Brit Toven-Lindsey, Erin M. Sparck, Kelly Kistner, Jacquelyn Ardam, Whitney Arnold
SPUR (2024) 7 (2): https://doi.org/10.18833/spur/7/2/2 Abstract:The benefits of undergraduate research experiences are well documented, yet few studies focus on programs designed to support students conducting research in the fields of humanities, arts, and social sciences. In this study, the authors examine learning experiences of students participating in the undergraduate research programs (URP) at UCLA, which support students conducting multiterm research or creative projects with faculty mentors. Drawing on data from students who participated in URP from 2015 to 2022 (N = 431), findings indicated that URP offered students structure, resources, mentorship, and peer networks to help them succeed. Further, students made significant gains in feeling connected to the research community and reported that undergraduate research was an important component of their academic career.
Toven-Lindsey, Brit, Erin M. Sparck, Kelly Kistner, Jacquelyn Ardam, Whitney Arnold. 2023. Undergraduate Research in Humanities,… - Commentary‐ Joseph J. Reczek
SPUR (2024) 7 (2): https://doi.org/10.18833/spur/7/2/11 Abstract:ConnectUR is a relatively new annual conference from the Council on Undergraduate Research that brings together all professional constituencies related to undergraduate research. This commentary offers context for the importance, potential impact, and evolutions of ConnectUR as the most inclusive and complete event for leaders in all areas related to undergraduate research.
Reczek, Joseph. 2023. Everything Undergraduate Research in One Place: A Commentary on the ConnectUR. Scholarship and…
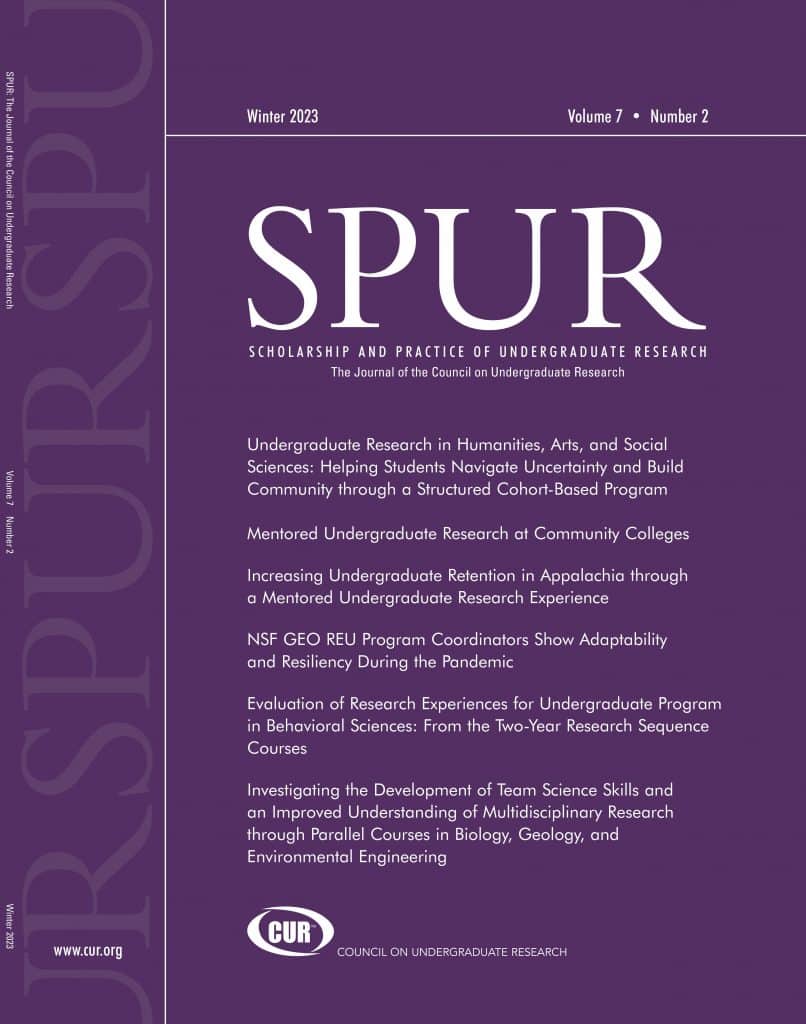